Research
The Hadlock Lab conducts translational research to improve risk models for clinical decision support and investigates novel methods to accelerate research discovery.
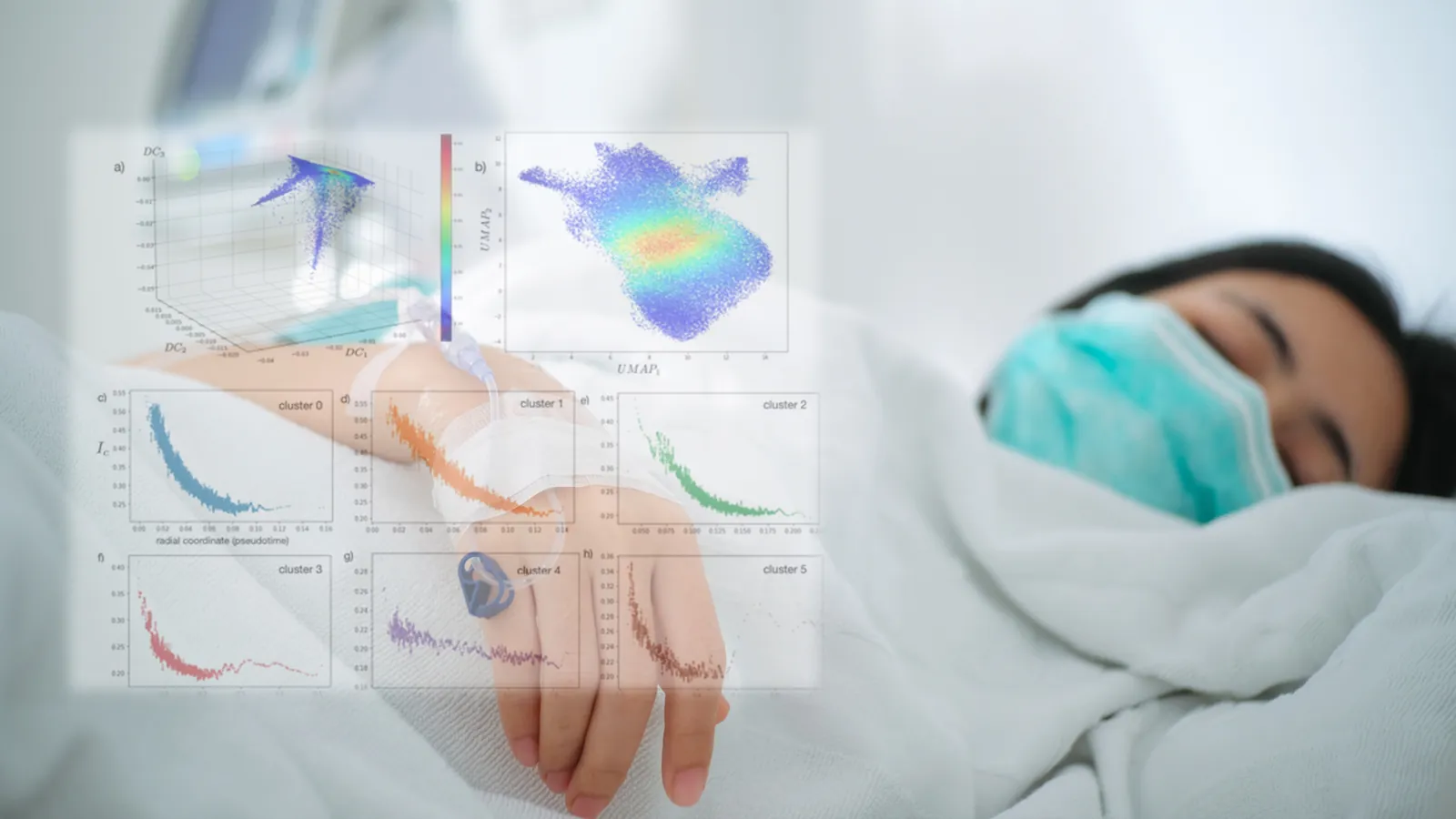
Research Overview
Our interdisciplinary lab is accelerating translational research by integrating clinical data into systems biology at scale. Our goal is to improve the lives of people with immune-mediated inflammatory diseases (IMIDs). IMIDs are clinically diverse conditions characterized by immune dysregulation, chronic inflammation and potential organ damage. IMIDs include ulcerative colitis, Crohn’s disease, rheumatoid arthritis, psoriasis, psoriatic arthritis, ankylosing spondylitis, multiple sclerosis, systemic lupus erythematosus, Sjögren’s syndrome and others, including numerous rare diseases. The collective estimated prevalence of IMIDs is 5 percent to 7 percent, and IMIDs are frequently underdiagnosed. Individuals may experience years of delay and long-term harm before diagnosis, and often try multiple treatments before finding which ones provide benefit. In addition, medications that work well at first may lose efficacy over time. To advance understanding of IMID transitions, we are integrating domain-agnostic computational methods, knowledge ontologies, and longitudinal data with genotype, phenotype, and exposures. Applying a systems biology approach, we do not limit research to high-level disease labels, but instead consider genotype and longitudinal patterns in both phenotype (such as multiomics, clinical observations and patient reported outcomes) and exposures (such as immunomodulatory medications, infectious disease and health-related social needs). We also investigate the intersection of IMIDs, aging and common chronic multimorbidities, which occur at a higher rate in the population of people with IMIDs. Through interdisciplinary collaboration, we aim for earlier IMID prediction, better personalized treatment and prevention of sequelae.
Research Focus
Our research focuses on two areas.
- Explainable predictive risk models for informing clinical decisions and surfacing new biomedical insights into mechanisms of disease.
- Domain-agnostic methods for accelerating discovery into mechanisms of disease.
Risk modeling
Our initial research has focused on real-world evidence from over 26 million electronic health records (EHRs) to inform decisions about patient care and research priorities. EHRs provide longitudinal data for large cohorts, which enable multivariate modeling across medical conditions, medications, and clinical phenotype observations – even when focusing on specific subpopulations. For example, with the COVID-19 pandemic, we investigated questions about pregnancy, aging, hypertension and anti-hypertensive drugs and developed risk-prediction models for COVID-19 integrating IMIDs, history of immunomodulatory drugs, vaccination status and additional chronic comorbidities. EHR data is vast (billions of observations), sensitive, and siloed, with significant barriers to even foundational levels of interoperability. In addition, the data is predominantly sparse, lacking in temporal alignment, and subject to significant ascertainment bias that varies over both time and space. We navigate with care to design models that will inform clinical decisions or research, and our assessments go beyond simple performance metrics to focus on reproducibility, explainability and biomedical relevance. Our lab has established productive collaborations with clinical experts in IMIDs (rheumatology, gastroenterology and neurology), infectious disease, obstetrics, gerontology and nephrology. These experts help us prioritize investigations that are of greatest importance for patient care, and understand where artifacts of current healthcare delivery and EHR documentation may reflect or mask underlying biomedical patterns. We have developed curated ontology-based, physician-reviewed human/computer-readable phenotype libraries for higher fidelity measures in areas essential for understanding IMIDs. This includes sharable human/computer-readable phenotypes with a focus on hierarchical definitions for IMID conditions, chronic multimorbidities, immunomodulatory medications, and features related to endothelial function and immune status. These phenotype libraries have also been valuable for collaboration with colleagues, investigating antivirals during acute COVID-19 and mortality, and integrating EHR data with prospective studies for deep-immunophenotyping (acute COVID-19 and post-acute sequelae (PASC). We are currently conducting research on data which combines retrospective real-world evidence with richer prospective data. For example, we are investigating IMIDs in the NIH All of Us Research Hub, which includes data from a diverse cohort: EHRs, genomics, social determinants of health, lifestyle information, physical measurements and wearables.
Domain agnostic methods
Our research includes both hypothesis discovery and traditional pharmacoepidemiology. As such, we evaluate and apply a wide range of existing and emerging methods in data science to improve the accuracy and trustworthiness of models, including multiple machine-learning methods, explainability and fairness algorithms, and both per-patient and geocoded census-tract level measures of social determinants of health. Currently, there are two areas where we are developing novel methods. One is work with the NIH NCATS Biomedical Data Translator Consortium, bridging the semantic gap between real-world clinical data and knowledge graphs. Our contribution is developing privacy-preserving knowledge graphs from real-world evidence for integration with multiomic knowledge graphs from other sources. Another is advances for addressing sparse, irregular and multivariate time series, a problem encountered frequently with both real-world evidence and clinical study data. This work includes data transformation, such as temporal synthetic minority oversampling, and alternate approaches to analyzing health trajectories over time.
Work at ISB
The Hadlock Lab welcomes data scientists and postdoctoral fellows.
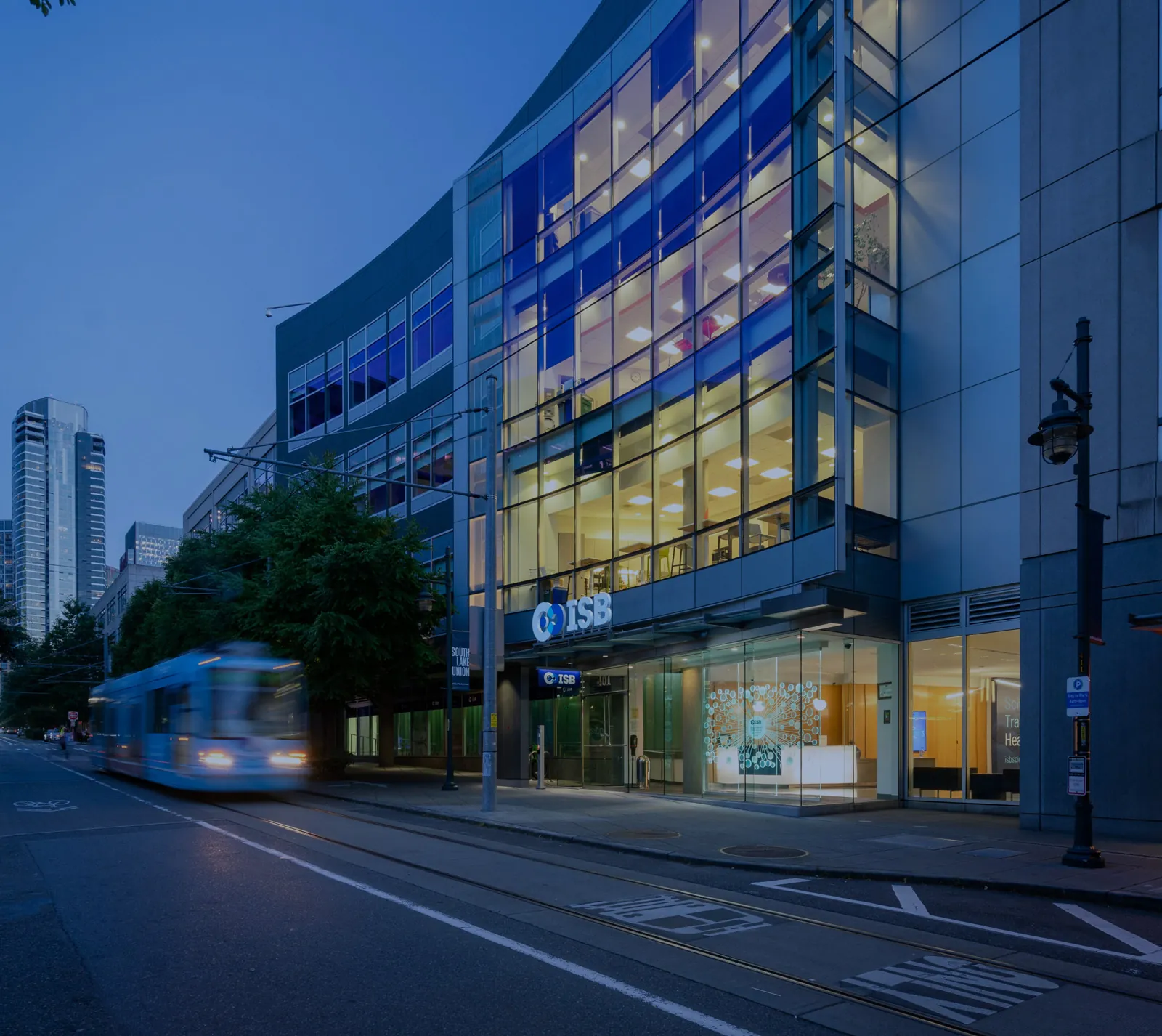